AI Tools for Predictive Maintenance: Revolutionizing Industrial Operations
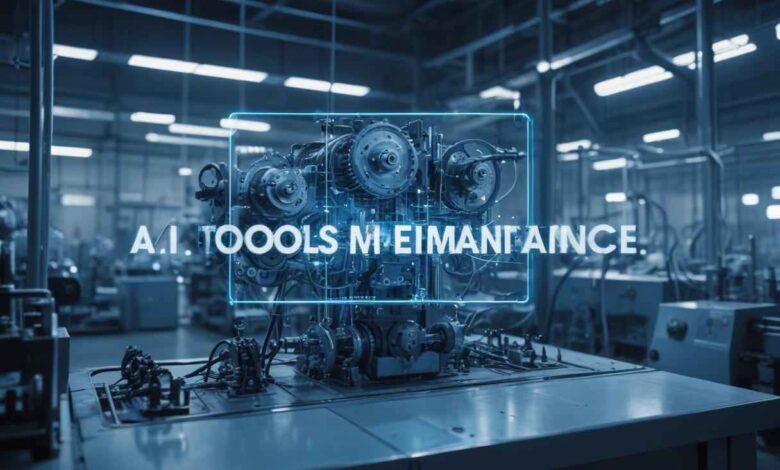
In the realm of industrial operations, two things are most important: efficiency and reliability. If you can forecast and forestall the failure of equipment, it can sharply lower downtime while shooting productivity skyward and saving costs. This is why artificial intelligence tools find their role in predictive maintenance and why they bring revolutionizing alternatives to common maintenance practices. Throughout this detailed manual, we will navigate through the universe of AI-fueled predictive maintenance—discussing its advantages without forgetting its use cases plus top market tools available for use.
Comprehending Predictive Maintenance
Predictive maintenance stands as an innovative way to the maintenance process that uses information and specialized analysis to foresee when equipment failure will most likely happen. AI algorithms can detect patterns and irregularities from historical data and sensor readings—signals that could lead to any future issues (through other relevant information). This allows the team of maintenance to step in before a breakdown happens; thereby, they can reduce loss due to unexpected downtime plus the potential value of asset life.
Explaining the Significance of Predictive Maintenance
Predictive maintenance has some major advantages over traditional reactive or scheduled maintenance. For one, it assists in preventing costly downtime by dealing with the issue before it becomes a major failure problem. Secondly, it fine-tunes maintenance calendars so that organizations undertake maintenance activities only when they need to, thus cutting down on unnecessary operational disruptions. Thirdly, it spots problems at their nascent stages which allows for rectification; hence it saves on the cost of premature replacements and helps in extending the lifespan of equipment. Maintenance costs can add up to 15-40% of direct operating costs— including spare parts and labor—to support systems when no predictive techniques are used during operation.
AI and Predictive Maintenance: IBM Watson IoT
1. IBM Watson IoT
IBM Watson IoT is considered a top-notch platform that provides a group of artificially intelligent tools for predictive maintenance. It involves using machine learning algorithms and advanced analytics in real-time to scrutinize huge volumes of sensor data. This allows it to detect abnormal patterns as they occur and thereby predict failure of equipment with a high degree of precision—empowering organizations to act proactively towards preventing downtimes and ensuring optimal maintenance workflows.
External Link: IBM Watson IoT
2. Microsoft Azure Machine Learning
Another potent offering in the realm of predictive maintenance is Microsoft Azure Machine Learning. By leveraging a diverse range of tools and algorithms within its framework, Azure Machine Learning has the capability to scrutinize data coming from different origins such as IoT devices and industrial sensors. On catching wind of possible issues at their nascence, it takes the liberty to arm stakeholders with actionable insights—not forgetting an optimization of maintenance schedules so that disruptions can be kept at bay.
External Link: Microsoft Azure Machine Learning
3. SAS Predictive Maintenance and Quality
SAS Predictive Maintenance and Quality is a one-stop comprehensive package. An application that is to guide firms in establishing the best maintenance tactics through their optimization. It utilizes predictive analytics, machine learning, and artificial intelligence to foretell when equipment failures will happen and detect what caused the problem—thus recommending what should be done as a result of it. Loss of time can be minimized by this tool; it also enhances asset reliability while increasing operational efficiency.
The Advantages of AI-Driven Predictive Maintenance
The advantages of AI-driven predictive maintenance are well-known, but the proper use of these tools cannot be achieved without due attention to careful planning and execution. Here are some key steps to consider:
1. Data Collection and Integration
The first step toward the adoption of AI tools for predictive maintenance is to gather a set of pertinent data sourced from every point deemed necessary. This could entail sensor data, logs of past maintenance operations, historical records on the performance data among others. Post collection, the data has to find a way into an integrated platform where all analyses will be carried out.
2. Model Training and Deployment
After data has been collected and consolidated, the immediate action would be to establish AI models for predictive maintenance. This includes choosing suitable algorithms, preprocessing data, and training models through historical records—an exercise that once completed demands constant vigilance plus fine-tuning of the model to better accuracy with time.
3. Deployment and Monitoring
The models should be deployed for production after being trained. Ensure close monitoring of model performance plus re-calibration upon any variations detected in order to guarantee accurate predictions; this is an essential consideration when it comes to real-time monitoring and prediction. Moreover, organizations must have action-based processes from the intelligence of these models—whether they schedule maintenance tasks or order replacement parts.
Understanding Predictive Maintenance
Predictive maintenance is an innovative approach that uses data and specialized analysis to predict when equipment failures are likely to occur. AI algorithms analyze historical data and sensor readings to detect patterns and anomalies that could signal future issues. This proactive approach allows maintenance teams to intervene before breakdowns occur, reducing downtime and extending asset life.
Exploring the Benefits of Predictive Maintenance
Predictive maintenance offers several advantages over reactive or scheduled maintenance. It helps prevent costly downtime by addressing issues before they escalate into major problems. It optimizes maintenance schedules, ensuring interventions only when necessary and minimizing operational disruptions. By identifying problems early, it also reduces the need for premature replacements, thereby extending equipment lifespan. Without predictive techniques, maintenance costs can contribute significantly—up to 15-40% of direct operating costs.
AI and Predictive Maintenance: Leading Platforms
1. IBM Watson IoT
IBM Watson IoT is a leading platform that leverages AI and machine learning to analyze large volumes of sensor data in real-time. It detects abnormal patterns and predicts equipment failures with high precision, empowering proactive maintenance workflows.
External Link: IBM Watson IoT
2. Microsoft Azure Machine Learning
Microsoft Azure Machine Learning offers a diverse set of tools and algorithms to analyze data from IoT devices and industrial sensors. It provides actionable insights and optimizes maintenance schedules to prevent disruptions.
External Link: Microsoft Azure Machine Learning
3. SAS Predictive Maintenance and Quality
SAS Predictive Maintenance and Quality integrates predictive analytics and AI to predict equipment failures and recommend optimal maintenance strategies. It enhances asset reliability and operational efficiency, minimizing downtime.
Implementing AI-Driven Predictive Maintenance
Effective implementation of AI-driven predictive maintenance requires careful planning and execution:
1. Data Collection and Integration
Collect relevant data sources such as sensor data, maintenance logs, and performance records. Integrate data into a unified platform for analysis.
2. Model Training and Deployment
Train AI models using historical data, select appropriate algorithms, and continuously fine-tune models for accuracy.
3. Deployment and Monitoring
Deploy trained models into production, monitor performance, and recalibrate as needed to ensure accurate predictions and proactive maintenance actions.
Key Considerations for Implementing AI-Driven Predictive Maintenance
Implementing AI-driven predictive maintenance involves several key considerations:
1. Data Collection and Integration
Begin by gathering comprehensive data from various sources including IoT devices, sensors, maintenance logs, and historical records. Integration of this data into a centralized platform is crucial for effective analysis.
2. Model Development and Training
Develop AI models tailored to predictive maintenance needs. This includes selecting appropriate algorithms, preprocessing data, and training models using historical data. Continuous refinement and tuning of these models ensure accuracy and reliability over time.
3. Deployment and Monitoring
Deploy trained models into production environments. Monitor their performance closely and implement mechanisms for real-time adjustments and recalibration as necessary. This proactive monitoring ensures that predictions remain accurate and actionable.
4. Integration with Maintenance Workflows
Integrate AI-driven insights seamlessly into existing maintenance workflows. This involves automating maintenance scheduling, predicting equipment failures, and recommending preemptive actions to minimize disruptions and optimize operational efficiency.
5. Continuous Improvement and Adaptation
AI-driven predictive maintenance is not a one-time implementation but a continuous process of improvement. Regularly assess and update AI models based on new data and evolving operational conditions to maintain effectiveness and relevance.
Final Thoughts
In conclusion, artificial intelligence in predictive maintenance represents a paradigm shift towards proactive and efficient industrial upkeep. By harnessing the power of AI algorithms and advanced analytics, organizations can anticipate equipment failures, reduce downtime, and maximize asset performance. Platforms like IBM Watson IoT, Microsoft Azure Machine Learning, and SAS Predictive Maintenance and Quality offer sophisticated tools to support these initiatives. However, successful implementation requires careful planning, robust data management, and a commitment to ongoing refinement. Embracing AI-driven predictive maintenance promises to revolutionize industrial operations, ensuring higher reliability, enhanced efficiency, and significant cost savings in the long term.
Conclusion
AI-driven predictive maintenance represents a transformative leap in industrial maintenance practices. By leveraging AI algorithms and data integration, organizations can predict and prevent equipment failures, minimize downtime, and optimize maintenance processes. Leading platforms like IBM Watson IoT, Microsoft Azure Machine Learning, and SAS Predictive Maintenance and Quality offer robust solutions. Success requires strategic planning, data integration, and ongoing monitoring. With the right approach, AI-driven predictive maintenance promises to enhance efficiency, reliability, and cost-effectiveness across industries.